mirror of
https://github.com/facebook/rocksdb.git
synced 2024-11-30 04:41:49 +00:00
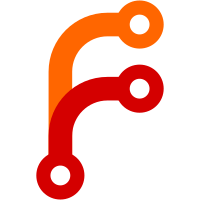
Summary: New experimental option BBTO::optimize_filters_for_memory builds filters that maximize their use of "usable size" from malloc_usable_size, which is also used to compute block cache charges. Rather than always "rounding up," we track state in the BloomFilterPolicy object to mix essentially "rounding down" and "rounding up" so that the average FP rate of all generated filters is the same as without the option. (YMMV as heavily accessed filters might be unluckily lower accuracy.) Thus, the option near-minimizes what the block cache considers as "memory used" for a given target Bloom filter false positive rate and Bloom filter implementation. There are no forward or backward compatibility issues with this change, though it only works on the format_version=5 Bloom filter. With Jemalloc, we see about 10% reduction in memory footprint (and block cache charge) for Bloom filters, but 1-2% increase in storage footprint, due to encoding efficiency losses (FP rate is non-linear with bits/key). Why not weighted random round up/down rather than state tracking? By only requiring malloc_usable_size, we don't actually know what the next larger and next smaller usable sizes for the allocator are. We pick a requested size, accept and use whatever usable size it has, and use the difference to inform our next choice. This allows us to narrow in on the right balance without tracking/predicting usable sizes. Why not weight history of generated filter false positive rates by number of keys? This could lead to excess skew in small filters after generating a large filter. Results from filter_bench with jemalloc (irrelevant details omitted): (normal keys/filter, but high variance) $ ./filter_bench -quick -impl=2 -average_keys_per_filter=30000 -vary_key_count_ratio=0.9 Build avg ns/key: 29.6278 Number of filters: 5516 Total size (MB): 200.046 Reported total allocated memory (MB): 220.597 Reported internal fragmentation: 10.2732% Bits/key stored: 10.0097 Average FP rate %: 0.965228 $ ./filter_bench -quick -impl=2 -average_keys_per_filter=30000 -vary_key_count_ratio=0.9 -optimize_filters_for_memory Build avg ns/key: 30.5104 Number of filters: 5464 Total size (MB): 200.015 Reported total allocated memory (MB): 200.322 Reported internal fragmentation: 0.153709% Bits/key stored: 10.1011 Average FP rate %: 0.966313 (very few keys / filter, optimization not as effective due to ~59 byte internal fragmentation in blocked Bloom filter representation) $ ./filter_bench -quick -impl=2 -average_keys_per_filter=1000 -vary_key_count_ratio=0.9 Build avg ns/key: 29.5649 Number of filters: 162950 Total size (MB): 200.001 Reported total allocated memory (MB): 224.624 Reported internal fragmentation: 12.3117% Bits/key stored: 10.2951 Average FP rate %: 0.821534 $ ./filter_bench -quick -impl=2 -average_keys_per_filter=1000 -vary_key_count_ratio=0.9 -optimize_filters_for_memory Build avg ns/key: 31.8057 Number of filters: 159849 Total size (MB): 200 Reported total allocated memory (MB): 208.846 Reported internal fragmentation: 4.42297% Bits/key stored: 10.4948 Average FP rate %: 0.811006 (high keys/filter) $ ./filter_bench -quick -impl=2 -average_keys_per_filter=1000000 -vary_key_count_ratio=0.9 Build avg ns/key: 29.7017 Number of filters: 164 Total size (MB): 200.352 Reported total allocated memory (MB): 221.5 Reported internal fragmentation: 10.5552% Bits/key stored: 10.0003 Average FP rate %: 0.969358 $ ./filter_bench -quick -impl=2 -average_keys_per_filter=1000000 -vary_key_count_ratio=0.9 -optimize_filters_for_memory Build avg ns/key: 30.7131 Number of filters: 160 Total size (MB): 200.928 Reported total allocated memory (MB): 200.938 Reported internal fragmentation: 0.00448054% Bits/key stored: 10.1852 Average FP rate %: 0.963387 And from db_bench (block cache) with jemalloc: $ ./db_bench -db=/dev/shm/dbbench.no_optimize -benchmarks=fillrandom -format_version=5 -value_size=90 -bloom_bits=10 -num=2000000 -threads=8 -compaction_style=2 -fifo_compaction_max_table_files_size_mb=10000 -fifo_compaction_allow_compaction=false $ ./db_bench -db=/dev/shm/dbbench -benchmarks=fillrandom -format_version=5 -value_size=90 -bloom_bits=10 -num=2000000 -threads=8 -optimize_filters_for_memory -compaction_style=2 -fifo_compaction_max_table_files_size_mb=10000 -fifo_compaction_allow_compaction=false $ (for FILE in /dev/shm/dbbench.no_optimize/*.sst; do ./sst_dump --file=$FILE --show_properties | grep 'filter block' ; done) | awk '{ t += $4; } END { print t; }' 17063835 $ (for FILE in /dev/shm/dbbench/*.sst; do ./sst_dump --file=$FILE --show_properties | grep 'filter block' ; done) | awk '{ t += $4; } END { print t; }' 17430747 $ #^ 2.1% additional filter storage $ ./db_bench -db=/dev/shm/dbbench.no_optimize -use_existing_db -benchmarks=readrandom,stats -statistics -bloom_bits=10 -num=2000000 -compaction_style=2 -fifo_compaction_max_table_files_size_mb=10000 -fifo_compaction_allow_compaction=false -duration=10 -cache_index_and_filter_blocks -cache_size=1000000000 rocksdb.block.cache.index.add COUNT : 33 rocksdb.block.cache.index.bytes.insert COUNT : 8440400 rocksdb.block.cache.filter.add COUNT : 33 rocksdb.block.cache.filter.bytes.insert COUNT : 21087528 rocksdb.bloom.filter.useful COUNT : 4963889 rocksdb.bloom.filter.full.positive COUNT : 1214081 rocksdb.bloom.filter.full.true.positive COUNT : 1161999 $ #^ 1.04 % observed FP rate $ ./db_bench -db=/dev/shm/dbbench -use_existing_db -benchmarks=readrandom,stats -statistics -bloom_bits=10 -num=2000000 -compaction_style=2 -fifo_compaction_max_table_files_size_mb=10000 -fifo_compaction_allow_compaction=false -optimize_filters_for_memory -duration=10 -cache_index_and_filter_blocks -cache_size=1000000000 rocksdb.block.cache.index.add COUNT : 33 rocksdb.block.cache.index.bytes.insert COUNT : 8448592 rocksdb.block.cache.filter.add COUNT : 33 rocksdb.block.cache.filter.bytes.insert COUNT : 18220328 rocksdb.bloom.filter.useful COUNT : 5360933 rocksdb.bloom.filter.full.positive COUNT : 1321315 rocksdb.bloom.filter.full.true.positive COUNT : 1262999 $ #^ 1.08 % observed FP rate, 13.6% less memory usage for filters (Due to specific key density, this example tends to generate filters that are "worse than average" for internal fragmentation. "Better than average" cases can show little or no improvement.) Pull Request resolved: https://github.com/facebook/rocksdb/pull/6427 Test Plan: unit test added, 'make check' with gcc, clang and valgrind Reviewed By: siying Differential Revision: D22124374 Pulled By: pdillinger fbshipit-source-id: f3e3aa152f9043ddf4fae25799e76341d0d8714e
771 lines
27 KiB
C++
771 lines
27 KiB
C++
// Copyright (c) 2011-present, Facebook, Inc. All rights reserved.
|
|
// This source code is licensed under both the GPLv2 (found in the
|
|
// COPYING file in the root directory) and Apache 2.0 License
|
|
// (found in the LICENSE.Apache file in the root directory).
|
|
|
|
#if !defined(GFLAGS) || defined(ROCKSDB_LITE)
|
|
#include <cstdio>
|
|
int main() {
|
|
fprintf(stderr, "filter_bench requires gflags and !ROCKSDB_LITE\n");
|
|
return 1;
|
|
}
|
|
#else
|
|
|
|
#include <cinttypes>
|
|
#include <iostream>
|
|
#include <sstream>
|
|
#include <vector>
|
|
|
|
#include "memory/arena.h"
|
|
#include "port/port.h"
|
|
#include "port/stack_trace.h"
|
|
#include "table/block_based/filter_policy_internal.h"
|
|
#include "table/block_based/full_filter_block.h"
|
|
#include "table/block_based/mock_block_based_table.h"
|
|
#include "table/plain/plain_table_bloom.h"
|
|
#include "util/cast_util.h"
|
|
#include "util/gflags_compat.h"
|
|
#include "util/hash.h"
|
|
#include "util/random.h"
|
|
#include "util/stderr_logger.h"
|
|
#include "util/stop_watch.h"
|
|
|
|
using GFLAGS_NAMESPACE::ParseCommandLineFlags;
|
|
using GFLAGS_NAMESPACE::RegisterFlagValidator;
|
|
using GFLAGS_NAMESPACE::SetUsageMessage;
|
|
|
|
DEFINE_uint32(seed, 0, "Seed for random number generators");
|
|
|
|
DEFINE_double(working_mem_size_mb, 200,
|
|
"MB of memory to get up to among all filters, unless "
|
|
"m_keys_total_max is specified.");
|
|
|
|
DEFINE_uint32(average_keys_per_filter, 10000,
|
|
"Average number of keys per filter");
|
|
|
|
DEFINE_double(vary_key_count_ratio, 0.4,
|
|
"Vary number of keys by up to +/- vary_key_count_ratio * "
|
|
"average_keys_per_filter.");
|
|
|
|
DEFINE_uint32(key_size, 24, "Average number of bytes for each key");
|
|
|
|
DEFINE_bool(vary_key_alignment, true,
|
|
"Whether to vary key alignment (default: at least 32-bit "
|
|
"alignment)");
|
|
|
|
DEFINE_uint32(vary_key_size_log2_interval, 5,
|
|
"Use same key size 2^n times, then change. Key size varies from "
|
|
"-2 to +2 bytes vs. average, unless n>=30 to fix key size.");
|
|
|
|
DEFINE_uint32(batch_size, 8, "Number of keys to group in each batch");
|
|
|
|
DEFINE_double(bits_per_key, 10.0, "Bits per key setting for filters");
|
|
|
|
DEFINE_double(m_queries, 200, "Millions of queries for each test mode");
|
|
|
|
DEFINE_double(m_keys_total_max, 0,
|
|
"Maximum total keys added to filters, in millions. "
|
|
"0 (default) disables. Non-zero overrides working_mem_size_mb "
|
|
"option.");
|
|
|
|
DEFINE_bool(use_full_block_reader, false,
|
|
"Use FullFilterBlockReader interface rather than FilterBitsReader");
|
|
|
|
DEFINE_bool(use_plain_table_bloom, false,
|
|
"Use PlainTableBloom structure and interface rather than "
|
|
"FilterBitsReader/FullFilterBlockReader");
|
|
|
|
DEFINE_bool(new_builder, false,
|
|
"Whether to create a new builder for each new filter");
|
|
|
|
DEFINE_uint32(impl, 0,
|
|
"Select filter implementation. Without -use_plain_table_bloom:"
|
|
"0 = full filter, 1 = block-based filter. With "
|
|
"-use_plain_table_bloom: 0 = no locality, 1 = locality.");
|
|
|
|
DEFINE_bool(net_includes_hashing, false,
|
|
"Whether query net ns/op times should include hashing. "
|
|
"(if not, dry run will include hashing) "
|
|
"(build times always include hashing)");
|
|
|
|
DEFINE_bool(optimize_filters_for_memory, false,
|
|
"Setting for BlockBasedTableOptions::optimize_filters_for_memory");
|
|
|
|
DEFINE_bool(quick, false, "Run more limited set of tests, fewer queries");
|
|
|
|
DEFINE_bool(best_case, false, "Run limited tests only for best-case");
|
|
|
|
DEFINE_bool(allow_bad_fp_rate, false, "Continue even if FP rate is bad");
|
|
|
|
DEFINE_bool(legend, false,
|
|
"Print more information about interpreting results instead of "
|
|
"running tests");
|
|
|
|
DEFINE_uint32(runs, 1, "Number of times to rebuild and run benchmark tests");
|
|
|
|
void _always_assert_fail(int line, const char *file, const char *expr) {
|
|
fprintf(stderr, "%s: %d: Assertion %s failed\n", file, line, expr);
|
|
abort();
|
|
}
|
|
|
|
#define ALWAYS_ASSERT(cond) \
|
|
((cond) ? (void)0 : ::_always_assert_fail(__LINE__, __FILE__, #cond))
|
|
|
|
#ifndef NDEBUG
|
|
// This could affect build times enough that we should not include it for
|
|
// accurate speed tests
|
|
#define PREDICT_FP_RATE
|
|
#endif
|
|
|
|
using ROCKSDB_NAMESPACE::Arena;
|
|
using ROCKSDB_NAMESPACE::BlockContents;
|
|
using ROCKSDB_NAMESPACE::BloomFilterPolicy;
|
|
using ROCKSDB_NAMESPACE::BloomHash;
|
|
using ROCKSDB_NAMESPACE::BuiltinFilterBitsBuilder;
|
|
using ROCKSDB_NAMESPACE::CachableEntry;
|
|
using ROCKSDB_NAMESPACE::EncodeFixed32;
|
|
using ROCKSDB_NAMESPACE::fastrange32;
|
|
using ROCKSDB_NAMESPACE::FilterBitsReader;
|
|
using ROCKSDB_NAMESPACE::FilterBuildingContext;
|
|
using ROCKSDB_NAMESPACE::FullFilterBlockReader;
|
|
using ROCKSDB_NAMESPACE::GetSliceHash;
|
|
using ROCKSDB_NAMESPACE::GetSliceHash64;
|
|
using ROCKSDB_NAMESPACE::Lower32of64;
|
|
using ROCKSDB_NAMESPACE::ParsedFullFilterBlock;
|
|
using ROCKSDB_NAMESPACE::PlainTableBloomV1;
|
|
using ROCKSDB_NAMESPACE::Random32;
|
|
using ROCKSDB_NAMESPACE::Slice;
|
|
using ROCKSDB_NAMESPACE::static_cast_with_check;
|
|
using ROCKSDB_NAMESPACE::StderrLogger;
|
|
using ROCKSDB_NAMESPACE::mock::MockBlockBasedTableTester;
|
|
|
|
struct KeyMaker {
|
|
KeyMaker(size_t avg_size)
|
|
: smallest_size_(avg_size -
|
|
(FLAGS_vary_key_size_log2_interval >= 30 ? 2 : 0)),
|
|
buf_size_(avg_size + 11), // pad to vary key size and alignment
|
|
buf_(new char[buf_size_]) {
|
|
memset(buf_.get(), 0, buf_size_);
|
|
assert(smallest_size_ > 8);
|
|
}
|
|
size_t smallest_size_;
|
|
size_t buf_size_;
|
|
std::unique_ptr<char[]> buf_;
|
|
|
|
// Returns a unique(-ish) key based on the given parameter values. Each
|
|
// call returns a Slice from the same buffer so previously returned
|
|
// Slices should be considered invalidated.
|
|
Slice Get(uint32_t filter_num, uint32_t val_num) {
|
|
size_t start = FLAGS_vary_key_alignment ? val_num % 4 : 0;
|
|
size_t len = smallest_size_;
|
|
if (FLAGS_vary_key_size_log2_interval < 30) {
|
|
// To get range [avg_size - 2, avg_size + 2]
|
|
// use range [smallest_size, smallest_size + 4]
|
|
len += fastrange32(
|
|
(val_num >> FLAGS_vary_key_size_log2_interval) * 1234567891, 5);
|
|
}
|
|
char * data = buf_.get() + start;
|
|
// Populate key data such that all data makes it into a key of at
|
|
// least 8 bytes. We also don't want all the within-filter key
|
|
// variance confined to a contiguous 32 bits, because then a 32 bit
|
|
// hash function can "cheat" the false positive rate by
|
|
// approximating a perfect hash.
|
|
EncodeFixed32(data, val_num);
|
|
EncodeFixed32(data + 4, filter_num + val_num);
|
|
// ensure clearing leftovers from different alignment
|
|
EncodeFixed32(data + 8, 0);
|
|
return Slice(data, len);
|
|
}
|
|
};
|
|
|
|
void PrintWarnings() {
|
|
#if defined(__GNUC__) && !defined(__OPTIMIZE__)
|
|
fprintf(stdout,
|
|
"WARNING: Optimization is disabled: benchmarks unnecessarily slow\n");
|
|
#endif
|
|
#ifndef NDEBUG
|
|
fprintf(stdout,
|
|
"WARNING: Assertions are enabled; benchmarks unnecessarily slow\n");
|
|
#endif
|
|
}
|
|
|
|
struct FilterInfo {
|
|
uint32_t filter_id_ = 0;
|
|
std::unique_ptr<const char[]> owner_;
|
|
Slice filter_;
|
|
uint32_t keys_added_ = 0;
|
|
std::unique_ptr<FilterBitsReader> reader_;
|
|
std::unique_ptr<FullFilterBlockReader> full_block_reader_;
|
|
std::unique_ptr<PlainTableBloomV1> plain_table_bloom_;
|
|
uint64_t outside_queries_ = 0;
|
|
uint64_t false_positives_ = 0;
|
|
};
|
|
|
|
enum TestMode {
|
|
kSingleFilter,
|
|
kBatchPrepared,
|
|
kBatchUnprepared,
|
|
kFiftyOneFilter,
|
|
kEightyTwentyFilter,
|
|
kRandomFilter,
|
|
};
|
|
|
|
static const std::vector<TestMode> allTestModes = {
|
|
kSingleFilter, kBatchPrepared, kBatchUnprepared,
|
|
kFiftyOneFilter, kEightyTwentyFilter, kRandomFilter,
|
|
};
|
|
|
|
static const std::vector<TestMode> quickTestModes = {
|
|
kSingleFilter,
|
|
kRandomFilter,
|
|
};
|
|
|
|
static const std::vector<TestMode> bestCaseTestModes = {
|
|
kSingleFilter,
|
|
};
|
|
|
|
const char *TestModeToString(TestMode tm) {
|
|
switch (tm) {
|
|
case kSingleFilter:
|
|
return "Single filter";
|
|
case kBatchPrepared:
|
|
return "Batched, prepared";
|
|
case kBatchUnprepared:
|
|
return "Batched, unprepared";
|
|
case kFiftyOneFilter:
|
|
return "Skewed 50% in 1%";
|
|
case kEightyTwentyFilter:
|
|
return "Skewed 80% in 20%";
|
|
case kRandomFilter:
|
|
return "Random filter";
|
|
}
|
|
return "Bad TestMode";
|
|
}
|
|
|
|
// Do just enough to keep some data dependence for the
|
|
// compiler / CPU
|
|
static uint32_t DryRunNoHash(Slice &s) {
|
|
uint32_t sz = static_cast<uint32_t>(s.size());
|
|
if (sz >= 4) {
|
|
return sz + s.data()[3];
|
|
} else {
|
|
return sz;
|
|
}
|
|
}
|
|
|
|
static uint32_t DryRunHash32(Slice &s) {
|
|
// Same perf characteristics as GetSliceHash()
|
|
return BloomHash(s);
|
|
}
|
|
|
|
static uint32_t DryRunHash64(Slice &s) {
|
|
return Lower32of64(GetSliceHash64(s));
|
|
}
|
|
|
|
struct FilterBench : public MockBlockBasedTableTester {
|
|
std::vector<KeyMaker> kms_;
|
|
std::vector<FilterInfo> infos_;
|
|
Random32 random_;
|
|
std::ostringstream fp_rate_report_;
|
|
Arena arena_;
|
|
StderrLogger stderr_logger_;
|
|
double m_queries_;
|
|
|
|
FilterBench()
|
|
: MockBlockBasedTableTester(new BloomFilterPolicy(
|
|
FLAGS_bits_per_key,
|
|
static_cast<BloomFilterPolicy::Mode>(FLAGS_impl))),
|
|
random_(FLAGS_seed),
|
|
m_queries_(0) {
|
|
for (uint32_t i = 0; i < FLAGS_batch_size; ++i) {
|
|
kms_.emplace_back(FLAGS_key_size < 8 ? 8 : FLAGS_key_size);
|
|
}
|
|
ioptions_.info_log = &stderr_logger_;
|
|
table_options_.optimize_filters_for_memory =
|
|
FLAGS_optimize_filters_for_memory;
|
|
}
|
|
|
|
void Go();
|
|
|
|
double RandomQueryTest(uint32_t inside_threshold, bool dry_run,
|
|
TestMode mode);
|
|
};
|
|
|
|
void FilterBench::Go() {
|
|
if (FLAGS_use_plain_table_bloom && FLAGS_use_full_block_reader) {
|
|
throw std::runtime_error(
|
|
"Can't combine -use_plain_table_bloom and -use_full_block_reader");
|
|
}
|
|
if (FLAGS_use_plain_table_bloom) {
|
|
if (FLAGS_impl > 1) {
|
|
throw std::runtime_error(
|
|
"-impl must currently be >= 0 and <= 1 for Plain table");
|
|
}
|
|
} else {
|
|
if (FLAGS_impl == 1) {
|
|
throw std::runtime_error(
|
|
"Block-based filter not currently supported by filter_bench");
|
|
}
|
|
if (FLAGS_impl > 2) {
|
|
throw std::runtime_error(
|
|
"-impl must currently be 0 or 2 for Block-based table");
|
|
}
|
|
}
|
|
|
|
if (FLAGS_vary_key_count_ratio < 0.0 || FLAGS_vary_key_count_ratio > 1.0) {
|
|
throw std::runtime_error("-vary_key_count_ratio must be >= 0.0 and <= 1.0");
|
|
}
|
|
|
|
// For example, average_keys_per_filter = 100, vary_key_count_ratio = 0.1.
|
|
// Varys up to +/- 10 keys. variance_range = 21 (generating value 0..20).
|
|
// variance_offset = 10, so value - offset average value is always 0.
|
|
const uint32_t variance_range =
|
|
1 + 2 * static_cast<uint32_t>(FLAGS_vary_key_count_ratio *
|
|
FLAGS_average_keys_per_filter);
|
|
const uint32_t variance_offset = variance_range / 2;
|
|
|
|
const std::vector<TestMode> &testModes =
|
|
FLAGS_best_case ? bestCaseTestModes
|
|
: FLAGS_quick ? quickTestModes : allTestModes;
|
|
|
|
m_queries_ = FLAGS_m_queries;
|
|
double working_mem_size_mb = FLAGS_working_mem_size_mb;
|
|
if (FLAGS_quick) {
|
|
m_queries_ /= 7.0;
|
|
} else if (FLAGS_best_case) {
|
|
m_queries_ /= 3.0;
|
|
working_mem_size_mb /= 10.0;
|
|
}
|
|
|
|
std::cout << "Building..." << std::endl;
|
|
|
|
std::unique_ptr<BuiltinFilterBitsBuilder> builder;
|
|
|
|
size_t total_memory_used = 0;
|
|
size_t total_size = 0;
|
|
size_t total_keys_added = 0;
|
|
#ifdef PREDICT_FP_RATE
|
|
double weighted_predicted_fp_rate = 0.0;
|
|
#endif
|
|
size_t max_total_keys;
|
|
size_t max_mem;
|
|
if (FLAGS_m_keys_total_max > 0) {
|
|
max_total_keys = static_cast<size_t>(1000000 * FLAGS_m_keys_total_max);
|
|
max_mem = SIZE_MAX;
|
|
} else {
|
|
max_total_keys = SIZE_MAX;
|
|
max_mem = static_cast<size_t>(1024 * 1024 * working_mem_size_mb);
|
|
}
|
|
|
|
ROCKSDB_NAMESPACE::StopWatchNano timer(ROCKSDB_NAMESPACE::Env::Default(),
|
|
true);
|
|
|
|
infos_.clear();
|
|
while ((working_mem_size_mb == 0 || total_size < max_mem) &&
|
|
total_keys_added < max_total_keys) {
|
|
uint32_t filter_id = random_.Next();
|
|
uint32_t keys_to_add = FLAGS_average_keys_per_filter +
|
|
fastrange32(random_.Next(), variance_range) -
|
|
variance_offset;
|
|
if (max_total_keys - total_keys_added < keys_to_add) {
|
|
keys_to_add = static_cast<uint32_t>(max_total_keys - total_keys_added);
|
|
}
|
|
infos_.emplace_back();
|
|
FilterInfo &info = infos_.back();
|
|
info.filter_id_ = filter_id;
|
|
info.keys_added_ = keys_to_add;
|
|
if (FLAGS_use_plain_table_bloom) {
|
|
info.plain_table_bloom_.reset(new PlainTableBloomV1());
|
|
info.plain_table_bloom_->SetTotalBits(
|
|
&arena_, static_cast<uint32_t>(keys_to_add * FLAGS_bits_per_key),
|
|
FLAGS_impl, 0 /*huge_page*/, nullptr /*logger*/);
|
|
for (uint32_t i = 0; i < keys_to_add; ++i) {
|
|
uint32_t hash = GetSliceHash(kms_[0].Get(filter_id, i));
|
|
info.plain_table_bloom_->AddHash(hash);
|
|
}
|
|
info.filter_ = info.plain_table_bloom_->GetRawData();
|
|
} else {
|
|
if (!builder) {
|
|
builder.reset(
|
|
static_cast_with_check<BuiltinFilterBitsBuilder>(GetBuilder()));
|
|
}
|
|
for (uint32_t i = 0; i < keys_to_add; ++i) {
|
|
builder->AddKey(kms_[0].Get(filter_id, i));
|
|
}
|
|
info.filter_ = builder->Finish(&info.owner_);
|
|
#ifdef PREDICT_FP_RATE
|
|
weighted_predicted_fp_rate +=
|
|
keys_to_add *
|
|
builder->EstimatedFpRate(keys_to_add, info.filter_.size());
|
|
#endif
|
|
if (FLAGS_new_builder) {
|
|
builder.reset();
|
|
}
|
|
info.reader_.reset(
|
|
table_options_.filter_policy->GetFilterBitsReader(info.filter_));
|
|
CachableEntry<ParsedFullFilterBlock> block(
|
|
new ParsedFullFilterBlock(table_options_.filter_policy.get(),
|
|
BlockContents(info.filter_)),
|
|
nullptr /* cache */, nullptr /* cache_handle */,
|
|
true /* own_value */);
|
|
info.full_block_reader_.reset(
|
|
new FullFilterBlockReader(table_.get(), std::move(block)));
|
|
}
|
|
total_size += info.filter_.size();
|
|
#ifdef ROCKSDB_MALLOC_USABLE_SIZE
|
|
total_memory_used +=
|
|
malloc_usable_size(const_cast<char *>(info.filter_.data()));
|
|
#endif // ROCKSDB_MALLOC_USABLE_SIZE
|
|
total_keys_added += keys_to_add;
|
|
}
|
|
|
|
uint64_t elapsed_nanos = timer.ElapsedNanos();
|
|
double ns = double(elapsed_nanos) / total_keys_added;
|
|
std::cout << "Build avg ns/key: " << ns << std::endl;
|
|
std::cout << "Number of filters: " << infos_.size() << std::endl;
|
|
std::cout << "Total size (MB): " << total_size / 1024.0 / 1024.0 << std::endl;
|
|
if (total_memory_used > 0) {
|
|
std::cout << "Reported total allocated memory (MB): "
|
|
<< total_memory_used / 1024.0 / 1024.0 << std::endl;
|
|
std::cout << "Reported internal fragmentation: "
|
|
<< (total_memory_used - total_size) * 100.0 / total_size << "%"
|
|
<< std::endl;
|
|
}
|
|
|
|
double bpk = total_size * 8.0 / total_keys_added;
|
|
std::cout << "Bits/key stored: " << bpk << std::endl;
|
|
#ifdef PREDICT_FP_RATE
|
|
std::cout << "Predicted FP rate %: "
|
|
<< 100.0 * (weighted_predicted_fp_rate / total_keys_added)
|
|
<< std::endl;
|
|
#endif
|
|
if (!FLAGS_quick && !FLAGS_best_case) {
|
|
double tolerable_rate = std::pow(2.0, -(bpk - 1.0) / (1.4 + bpk / 50.0));
|
|
std::cout << "Best possible FP rate %: " << 100.0 * std::pow(2.0, -bpk)
|
|
<< std::endl;
|
|
std::cout << "Tolerable FP rate %: " << 100.0 * tolerable_rate << std::endl;
|
|
|
|
std::cout << "----------------------------" << std::endl;
|
|
std::cout << "Verifying..." << std::endl;
|
|
|
|
uint32_t outside_q_per_f =
|
|
static_cast<uint32_t>(m_queries_ * 1000000 / infos_.size());
|
|
uint64_t fps = 0;
|
|
for (uint32_t i = 0; i < infos_.size(); ++i) {
|
|
FilterInfo &info = infos_[i];
|
|
for (uint32_t j = 0; j < info.keys_added_; ++j) {
|
|
if (FLAGS_use_plain_table_bloom) {
|
|
uint32_t hash = GetSliceHash(kms_[0].Get(info.filter_id_, j));
|
|
ALWAYS_ASSERT(info.plain_table_bloom_->MayContainHash(hash));
|
|
} else {
|
|
ALWAYS_ASSERT(
|
|
info.reader_->MayMatch(kms_[0].Get(info.filter_id_, j)));
|
|
}
|
|
}
|
|
for (uint32_t j = 0; j < outside_q_per_f; ++j) {
|
|
if (FLAGS_use_plain_table_bloom) {
|
|
uint32_t hash =
|
|
GetSliceHash(kms_[0].Get(info.filter_id_, j | 0x80000000));
|
|
fps += info.plain_table_bloom_->MayContainHash(hash);
|
|
} else {
|
|
fps += info.reader_->MayMatch(
|
|
kms_[0].Get(info.filter_id_, j | 0x80000000));
|
|
}
|
|
}
|
|
}
|
|
std::cout << " No FNs :)" << std::endl;
|
|
double prelim_rate = double(fps) / outside_q_per_f / infos_.size();
|
|
std::cout << " Prelim FP rate %: " << (100.0 * prelim_rate) << std::endl;
|
|
|
|
if (!FLAGS_allow_bad_fp_rate) {
|
|
ALWAYS_ASSERT(prelim_rate < tolerable_rate);
|
|
}
|
|
}
|
|
|
|
std::cout << "----------------------------" << std::endl;
|
|
std::cout << "Mixed inside/outside queries..." << std::endl;
|
|
// 50% each inside and outside
|
|
uint32_t inside_threshold = UINT32_MAX / 2;
|
|
for (TestMode tm : testModes) {
|
|
random_.Seed(FLAGS_seed + 1);
|
|
double f = RandomQueryTest(inside_threshold, /*dry_run*/ false, tm);
|
|
random_.Seed(FLAGS_seed + 1);
|
|
double d = RandomQueryTest(inside_threshold, /*dry_run*/ true, tm);
|
|
std::cout << " " << TestModeToString(tm) << " net ns/op: " << (f - d)
|
|
<< std::endl;
|
|
}
|
|
|
|
if (!FLAGS_quick) {
|
|
std::cout << "----------------------------" << std::endl;
|
|
std::cout << "Inside queries (mostly)..." << std::endl;
|
|
// Do about 95% inside queries rather than 100% so that branch predictor
|
|
// can't give itself an artifically crazy advantage.
|
|
inside_threshold = UINT32_MAX / 20 * 19;
|
|
for (TestMode tm : testModes) {
|
|
random_.Seed(FLAGS_seed + 1);
|
|
double f = RandomQueryTest(inside_threshold, /*dry_run*/ false, tm);
|
|
random_.Seed(FLAGS_seed + 1);
|
|
double d = RandomQueryTest(inside_threshold, /*dry_run*/ true, tm);
|
|
std::cout << " " << TestModeToString(tm) << " net ns/op: " << (f - d)
|
|
<< std::endl;
|
|
}
|
|
|
|
std::cout << "----------------------------" << std::endl;
|
|
std::cout << "Outside queries (mostly)..." << std::endl;
|
|
// Do about 95% outside queries rather than 100% so that branch predictor
|
|
// can't give itself an artifically crazy advantage.
|
|
inside_threshold = UINT32_MAX / 20;
|
|
for (TestMode tm : testModes) {
|
|
random_.Seed(FLAGS_seed + 2);
|
|
double f = RandomQueryTest(inside_threshold, /*dry_run*/ false, tm);
|
|
random_.Seed(FLAGS_seed + 2);
|
|
double d = RandomQueryTest(inside_threshold, /*dry_run*/ true, tm);
|
|
std::cout << " " << TestModeToString(tm) << " net ns/op: " << (f - d)
|
|
<< std::endl;
|
|
}
|
|
}
|
|
std::cout << fp_rate_report_.str();
|
|
|
|
std::cout << "----------------------------" << std::endl;
|
|
std::cout << "Done. (For more info, run with -legend or -help.)" << std::endl;
|
|
}
|
|
|
|
double FilterBench::RandomQueryTest(uint32_t inside_threshold, bool dry_run,
|
|
TestMode mode) {
|
|
for (auto &info : infos_) {
|
|
info.outside_queries_ = 0;
|
|
info.false_positives_ = 0;
|
|
}
|
|
|
|
auto dry_run_hash_fn = DryRunNoHash;
|
|
if (!FLAGS_net_includes_hashing) {
|
|
if (FLAGS_impl < 2 || FLAGS_use_plain_table_bloom) {
|
|
dry_run_hash_fn = DryRunHash32;
|
|
} else {
|
|
dry_run_hash_fn = DryRunHash64;
|
|
}
|
|
}
|
|
|
|
uint32_t num_infos = static_cast<uint32_t>(infos_.size());
|
|
uint32_t dry_run_hash = 0;
|
|
uint64_t max_queries = static_cast<uint64_t>(m_queries_ * 1000000 + 0.50);
|
|
// Some filters may be considered secondary in order to implement skewed
|
|
// queries. num_primary_filters is the number that are to be treated as
|
|
// equal, and any remainder will be treated as secondary.
|
|
uint32_t num_primary_filters = num_infos;
|
|
// The proportion (when divided by 2^32 - 1) of filter queries going to
|
|
// the primary filters (default = all). The remainder of queries are
|
|
// against secondary filters.
|
|
uint32_t primary_filter_threshold = 0xffffffff;
|
|
if (mode == kSingleFilter) {
|
|
// 100% of queries to 1 filter
|
|
num_primary_filters = 1;
|
|
} else if (mode == kFiftyOneFilter) {
|
|
// 50% of queries
|
|
primary_filter_threshold /= 2;
|
|
// to 1% of filters
|
|
num_primary_filters = (num_primary_filters + 99) / 100;
|
|
} else if (mode == kEightyTwentyFilter) {
|
|
// 80% of queries
|
|
primary_filter_threshold = primary_filter_threshold / 5 * 4;
|
|
// to 20% of filters
|
|
num_primary_filters = (num_primary_filters + 4) / 5;
|
|
}
|
|
uint32_t batch_size = 1;
|
|
std::unique_ptr<Slice[]> batch_slices;
|
|
std::unique_ptr<Slice *[]> batch_slice_ptrs;
|
|
std::unique_ptr<bool[]> batch_results;
|
|
if (mode == kBatchPrepared || mode == kBatchUnprepared) {
|
|
batch_size = static_cast<uint32_t>(kms_.size());
|
|
}
|
|
|
|
batch_slices.reset(new Slice[batch_size]);
|
|
batch_slice_ptrs.reset(new Slice *[batch_size]);
|
|
batch_results.reset(new bool[batch_size]);
|
|
for (uint32_t i = 0; i < batch_size; ++i) {
|
|
batch_results[i] = false;
|
|
batch_slice_ptrs[i] = &batch_slices[i];
|
|
}
|
|
|
|
ROCKSDB_NAMESPACE::StopWatchNano timer(ROCKSDB_NAMESPACE::Env::Default(),
|
|
true);
|
|
|
|
for (uint64_t q = 0; q < max_queries; q += batch_size) {
|
|
bool inside_this_time = random_.Next() <= inside_threshold;
|
|
|
|
uint32_t filter_index;
|
|
if (random_.Next() <= primary_filter_threshold) {
|
|
filter_index = random_.Uniformish(num_primary_filters);
|
|
} else {
|
|
// secondary
|
|
filter_index = num_primary_filters +
|
|
random_.Uniformish(num_infos - num_primary_filters);
|
|
}
|
|
FilterInfo &info = infos_[filter_index];
|
|
for (uint32_t i = 0; i < batch_size; ++i) {
|
|
if (inside_this_time) {
|
|
batch_slices[i] =
|
|
kms_[i].Get(info.filter_id_, random_.Uniformish(info.keys_added_));
|
|
} else {
|
|
batch_slices[i] =
|
|
kms_[i].Get(info.filter_id_, random_.Uniformish(info.keys_added_) |
|
|
uint32_t{0x80000000});
|
|
info.outside_queries_++;
|
|
}
|
|
}
|
|
// TODO: implement batched interface to full block reader
|
|
// TODO: implement batched interface to plain table bloom
|
|
if (mode == kBatchPrepared && !FLAGS_use_full_block_reader &&
|
|
!FLAGS_use_plain_table_bloom) {
|
|
for (uint32_t i = 0; i < batch_size; ++i) {
|
|
batch_results[i] = false;
|
|
}
|
|
if (dry_run) {
|
|
for (uint32_t i = 0; i < batch_size; ++i) {
|
|
batch_results[i] = true;
|
|
dry_run_hash += dry_run_hash_fn(batch_slices[i]);
|
|
}
|
|
} else {
|
|
info.reader_->MayMatch(batch_size, batch_slice_ptrs.get(),
|
|
batch_results.get());
|
|
}
|
|
for (uint32_t i = 0; i < batch_size; ++i) {
|
|
if (inside_this_time) {
|
|
ALWAYS_ASSERT(batch_results[i]);
|
|
} else {
|
|
info.false_positives_ += batch_results[i];
|
|
}
|
|
}
|
|
} else {
|
|
for (uint32_t i = 0; i < batch_size; ++i) {
|
|
bool may_match;
|
|
if (FLAGS_use_plain_table_bloom) {
|
|
if (dry_run) {
|
|
dry_run_hash += dry_run_hash_fn(batch_slices[i]);
|
|
may_match = true;
|
|
} else {
|
|
uint32_t hash = GetSliceHash(batch_slices[i]);
|
|
may_match = info.plain_table_bloom_->MayContainHash(hash);
|
|
}
|
|
} else if (FLAGS_use_full_block_reader) {
|
|
if (dry_run) {
|
|
dry_run_hash += dry_run_hash_fn(batch_slices[i]);
|
|
may_match = true;
|
|
} else {
|
|
may_match = info.full_block_reader_->KeyMayMatch(
|
|
batch_slices[i],
|
|
/*prefix_extractor=*/nullptr,
|
|
/*block_offset=*/ROCKSDB_NAMESPACE::kNotValid,
|
|
/*no_io=*/false, /*const_ikey_ptr=*/nullptr,
|
|
/*get_context=*/nullptr,
|
|
/*lookup_context=*/nullptr);
|
|
}
|
|
} else {
|
|
if (dry_run) {
|
|
dry_run_hash += dry_run_hash_fn(batch_slices[i]);
|
|
may_match = true;
|
|
} else {
|
|
may_match = info.reader_->MayMatch(batch_slices[i]);
|
|
}
|
|
}
|
|
if (inside_this_time) {
|
|
ALWAYS_ASSERT(may_match);
|
|
} else {
|
|
info.false_positives_ += may_match;
|
|
}
|
|
}
|
|
}
|
|
}
|
|
|
|
uint64_t elapsed_nanos = timer.ElapsedNanos();
|
|
double ns = double(elapsed_nanos) / max_queries;
|
|
|
|
if (!FLAGS_quick) {
|
|
if (dry_run) {
|
|
// Printing part of hash prevents dry run components from being optimized
|
|
// away by compiler
|
|
std::cout << " Dry run (" << std::hex << (dry_run_hash & 0xfffff)
|
|
<< std::dec << ") ";
|
|
} else {
|
|
std::cout << " Gross filter ";
|
|
}
|
|
std::cout << "ns/op: " << ns << std::endl;
|
|
}
|
|
|
|
if (!dry_run) {
|
|
fp_rate_report_.str("");
|
|
uint64_t q = 0;
|
|
uint64_t fp = 0;
|
|
double worst_fp_rate = 0.0;
|
|
double best_fp_rate = 1.0;
|
|
for (auto &info : infos_) {
|
|
q += info.outside_queries_;
|
|
fp += info.false_positives_;
|
|
if (info.outside_queries_ > 0) {
|
|
double fp_rate = double(info.false_positives_) / info.outside_queries_;
|
|
worst_fp_rate = std::max(worst_fp_rate, fp_rate);
|
|
best_fp_rate = std::min(best_fp_rate, fp_rate);
|
|
}
|
|
}
|
|
fp_rate_report_ << " Average FP rate %: " << 100.0 * fp / q << std::endl;
|
|
if (!FLAGS_quick && !FLAGS_best_case) {
|
|
fp_rate_report_ << " Worst FP rate %: " << 100.0 * worst_fp_rate
|
|
<< std::endl;
|
|
fp_rate_report_ << " Best FP rate %: " << 100.0 * best_fp_rate
|
|
<< std::endl;
|
|
fp_rate_report_ << " Best possible bits/key: "
|
|
<< -std::log(double(fp) / q) / std::log(2.0) << std::endl;
|
|
}
|
|
}
|
|
return ns;
|
|
}
|
|
|
|
int main(int argc, char **argv) {
|
|
ROCKSDB_NAMESPACE::port::InstallStackTraceHandler();
|
|
SetUsageMessage(std::string("\nUSAGE:\n") + std::string(argv[0]) +
|
|
" [-quick] [OTHER OPTIONS]...");
|
|
ParseCommandLineFlags(&argc, &argv, true);
|
|
|
|
PrintWarnings();
|
|
|
|
if (FLAGS_legend) {
|
|
std::cout
|
|
<< "Legend:" << std::endl
|
|
<< " \"Inside\" - key that was added to filter" << std::endl
|
|
<< " \"Outside\" - key that was not added to filter" << std::endl
|
|
<< " \"FN\" - false negative query (must not happen)" << std::endl
|
|
<< " \"FP\" - false positive query (OK at low rate)" << std::endl
|
|
<< " \"Dry run\" - cost of testing and hashing overhead." << std::endl
|
|
<< " \"Gross filter\" - cost of filter queries including testing "
|
|
<< "\n and hashing overhead." << std::endl
|
|
<< " \"net\" - best estimate of time in filter operation, without "
|
|
<< "\n testing and hashing overhead (gross filter - dry run)"
|
|
<< std::endl
|
|
<< " \"ns/op\" - nanoseconds per operation (key query or add)"
|
|
<< std::endl
|
|
<< " \"Single filter\" - essentially minimum cost, assuming filter"
|
|
<< "\n fits easily in L1 CPU cache." << std::endl
|
|
<< " \"Batched, prepared\" - several queries at once against a"
|
|
<< "\n randomly chosen filter, using multi-query interface."
|
|
<< std::endl
|
|
<< " \"Batched, unprepared\" - similar, but using serial calls"
|
|
<< "\n to single query interface." << std::endl
|
|
<< " \"Random filter\" - a filter is chosen at random as target"
|
|
<< "\n of each query." << std::endl
|
|
<< " \"Skewed X% in Y%\" - like \"Random filter\" except Y% of"
|
|
<< "\n the filters are designated as \"hot\" and receive X%"
|
|
<< "\n of queries." << std::endl;
|
|
} else {
|
|
FilterBench b;
|
|
for (uint32_t i = 0; i < FLAGS_runs; ++i) {
|
|
b.Go();
|
|
FLAGS_seed += 100;
|
|
b.random_.Seed(FLAGS_seed);
|
|
}
|
|
}
|
|
|
|
return 0;
|
|
}
|
|
|
|
#endif // !defined(GFLAGS) || defined(ROCKSDB_LITE)
|